The rapid introduction of new medical devices and advanced diagnostic methods, together with genomics and patient-handled wearables, has started to generate vast amounts of data. Combined with new self-improving algorithms based on AI, this data represents a goldmine for achieving high-quality care for patients and increasing efficiencies in our healthcare systems.
But most healthcare systems are not yet ready since they do not have the foundation for managing and making use of the data available. Hospital CIOs need to act now in order to leverage the future possibilities that AI offers healthcare. In this article, Rachel Dunscombe shares her thoughts on how CIOs can build such a foundation to ensure data can be stored and handled appropriately, and how it can be made useful to physicians in their efforts to improve patient care—today and in the future.
I would like to encourage every CIO to be involved in creating the future. We will shape the future and we need to be brave. Don’t be afraid to be a pioneer.
Building the foundation
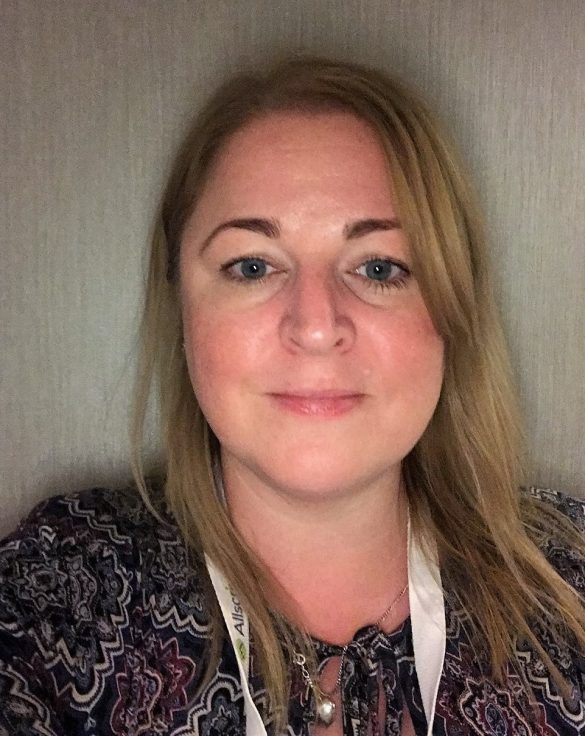
Rachel Dunscombe has extensive experience from various roles in healthcare IT, working with digital technologies, AI, standards and data management.
Standardization of data
The first step in making use of data is to store and handle it in a structured format. Rachel initiates our discussion by explaining that this is necessary in order to take advantage of AI in a future.
“To make the data useful for algorithms, you should move whatever possible to codification such as SNOMED CT or LOINC or similar,” Rachel explains. “We can do that by generating structured data directly from the IT systems we use in healthcare or applying smart NLP (Natural Language Processing) that can create structured summaries.”
According to Rachel, imaging is a great place to start because the quality of data is less variable and because imaging systems are designed to handle structured summaries and big data volumes, in contrast to, for example, EMRs. She mentions one specific area to begin with: “A good place is to start with structured data is to ensure there is a SNOMED-based summary of comorbidities for each patient in the enterprise imaging or PACS system.” This would lead to an enhanced patient overview for the radiologist without having to go through all previous reports.
A lot of what we want to do in the future relies on having access to a much cleaner and completer set of data. This is the foundation we need to start to build if we want to truly benefit from AI.
Clean and complete data
Rachel describes creating complete data as an immense and time-consuming housekeeping job for many hospitals today, and it will only get worse if no action is taken. “A lot of what we want to do in the future relies on having access to a much cleaner and completer set of data,” she says. “This is the foundation we need to start to build if we want to truly benefit from AI.”
Rachel tells us that there is some research from the U.S. on how to improve the quality of data, and she mentions the usability as a key puzzle piece. The system must allow the physicians in practice to put in the right data as part of their care process and create incentives to add that data.
She continues: “We need to define the minimum viable data for safe clinical care, and at the same time make it easy for physicians to understand how data improves the quality of patient care, without creating an overhead that reduces efficiency.”
Start designing data systemically
Rachel highlights the importance of establishing systemic design and systemic enterprise architecture, which looks at the provenance of data including its primary use of collection and its systemic use over time. “Users of the systems need to understand where the data initially was collected and where that data is used over the longitudinal journey of the patient. This is something that is not done today,” Rachel says.
“Data that is collected in one place may be used 25 years later in another place for another use. What is really important is to understand how and where that data was collected, the use it had at the time, and what confidence we can place in that data.”
A part of systemic design is to start to record the confidence in the data collected. Rachel clarifies with an example: “The confidence of a blood pressure measurement by the patient using a home device may be a medium level of confidence, but if it is recorded over a 12-hour period with a medical device in a hospital it may have high confidence.”
Systemic design is important to establish because when this data interacts with algorithms, decision making will be based on the level of confidence, which in turn is based on the provenance of that data. Rachel concludes: “The orchestration of data will be the big job for the future.”
Patient-generated data
Data generated by patients themselves is a concern for many CIOs. “Sooner or later we need to start to gather patient-generated data,” Rachel says. “We can’t ignore all these data types, and we need to look at which data is important, its confidence, and how we can use it in decision making.”
She says that an initial criterion for assessing which patient-generated data qualifies for storage in our healthcare systems might be based on whether or not it has been created using an approved medical device.
Rachel continues by sharing her experiences from the imaging scene, which she says lies at the forefront of utilizing patient-generated data: “We are already seeing a trend of patients taking pictures of wounds, skin disease, videos of walking, or recordings of children having seizures. Even now this can significantly help clinicians and their patients and there is some very valuable data we can gain by obtaining this from patients. However, there is also some data we won’t benefit from, and we need to establish standards to ensure that we only store data that falls in line with clinically generated data.”
The EMR will never own the genomics data because of its size. […] Putting big data or images in the EMR slows the system down because it is not designed for that type of data.
Genomic data
When discussing big data, we cannot avoid touching on the genome. Rachel admits that she has quite a controversial view on this topic and she sees that the genomics revolution on the consumer side is running faster than on the healthcare system side.
“Although the UK is a world leader in storing genomic data through Genomics England, it is not available to the public or all of the healthcare system,” she explains. “What will happen is that as genome sequencing prices decrease, the public will make its own genome tests and have bioinformatics summaries done themselves or by buying services from life science companies. That data will then be pushed back from their own record into the system.”
“Governments have underestimated the public’s desire to interact with genomics information. There is a great need for patients with long-term conditions such as Alzheimer’s, rare diseases or cancer to actually own that information.”
On the question of which IT system(s) should store the genome, Rachel has a very clear opinion: “The EMR will never own the genomics data because of its size.” She says that if there is one thing she has learned about the infrastructure from the EMRs, it is not to enter big data or images. “The entire EMR is designed to handle small fields of structured data. The genome on the other hand is by nature unstructured, big and complex, and should be stored in a vendor-neutral and standardized format in the vendor-neutral archive. A summary of the genotype and phenotype should then be pushed back to the EMR. Putting big data or images in the EMR slows the system down because it is not designed for that type of data.”
The integration between the PACS and the EMR will be all about creating a seamless integration between radiology images, videos, digital pathology with the electronic medical record. For usability and clinical safety, these systems need to be very closely linked together and feel systemically correct for the user.
Start working closer to the clinicians
Another piece of advice that Rachel offers is for the CIO to start working more closely with clinicians to help increase the usability of systems and create an understanding of what data is valuable and in what context. She mentions this as a prerequisite to encourage physicians to provide high-quality and complete data.
Rachel continues on the track of usability and how it can be enhanced by ensuring high interoperability: “Today we have many systems that need to exchange data with each other, and that will not change. We will continue to have many systems as new types of devices emerge all the time.”
She mentions the EMR and the PACS as two core systems that need to integrate smoothly with each other: “The integration between the PACS and the EMR will be all about creating a seamless integration between radiology images, videos, digital pathology with the electronic medical record,” she says. “For usability and clinical safety, these systems need to be very closely linked together and feel systemically correct for the user.”
As an example, Rachel mentions the multi-disciplinary team (MDT) meeting scenario: “If as a physician you participate in an MDT, you want access to the CT, lab results, the digital pathology images and so on. And you want these to be rendered in a context where they make sense. It will all be about optimizing the view of this information to ensure the best possible decision making.” Rachel says that the system itself should propose a “blended and context matched” set of information and only show relevant pieces for decision making.
When asked how healthcare could practically realize such interoperability, Rachel addresses web-based technologies. “Protocols such as FHIR can make real-time calls. The nice thing is that the system rapidly can call small pieces of data, instead of taking monolithically big HL7 messages.” She further explains that with each click, the system will call a small subset of the patient data, providing physicians with the information they need. Rachel calls this “every-click interoperability.”
Making use of data with AI
Rachel sits on the UK government’s AI committee and is heavily involved in the formulation of the UK’s AI strategy in healthcare. “We need to take away the ‘buzziness’ of AI, demystify it and look at it logically. Healthcare has used algorithms for many years in, for example, our labs to analyze blood and counting cells. What is different now is that we are moving toward self-modifying systems.”
She underlines that one of the challenges for the CIO will be to help physicians do the homework of understanding how the systems learn: “We need to adopt standards that allow physicians to look into the black boxes of AI, mainly for safety reasons. We also need to note how decisions are made and control sign-off in a standardized way to ensure safety so as to control to some extent what happens in these black boxes. The new way of medicine will be how to create, improve, and ensure the safety of algorithms.”
Rachel once again brings up imaging—and radiology and pathology in particular—as the first areas to start implementing AI, mainly because of the low variability in data quality and access to systems that can already handle big data and have started to incorporate algorithms in the workflow.
Rachel is firmly convinced that AI is no different from other technologies, that it is “just another tool” that will be necessary to truly make use of all the data we will keep in our future systems.
Summary of advice to the CIOs of the future
We are in the midst of a data explosion. Patient-generated and genomics data will play a key role in the future of medicine, and together with new AI tools and algorithms, it gives endless opportunities to healthcare. But today’s healthcare systems are not ready. It is up to each and every CIO to set up a strategy and start building an infrastructure and foundation to handle this data and make sure physicians and patients can benefit from it.
A first step to create this foundation is to ensure clean, complete and high-quality data. This is mainly done by standardizing data, confirming high usability, and establishing close integration between systems. Healthcare IT systems should be systemically designed to correctly orchestrate data, and provide information about where and when it was collected, how it initially was used, and enable longitudinal use of data. As Rachel describes it: “Data is for life, not one time.”
In terms of making use of data, AI will play a key role. CIOs need to work closely with physicians to understand what and when data is valuable in the clinical context, and assist physicians to understand how AI will improve over time. Only then can data and AI provide us with a safe and valuable toolset to improve healthcare as we know it.
Rachel offers a final piece of advice: “There do not have answers to all the questions yet. I would like to encourage every CIO to be involved in creating the future. We will shape the future and we need to be brave. We live in a space where we cannot know everything because it is new. Don’t be afraid to be a pioneer.”